What Are the Challenges in Developing Explainable AI?
Another significant challenge is the balance between model complexity and interpretability. More complex models generally provide higher accuracy but tend to be less interpretable. On the other hand, simpler models are easier to interpret but may sacrifice predictive performance. Finding the sweet spot where AI systems are both accurate and explainable is akin to walking a tightrope between innovation and practicality.
Moreover, ensuring fairness and avoiding bias in AI models is crucial. Biases in data or algorithms can lead to unjust or discriminatory outcomes, which is particularly concerning in sensitive domains like hiring or criminal justice. Developing methods to detect and mitigate biases while maintaining explainability is a critical frontier in AI ethics and governance.
Technological limitations also play a role. Current methods for explaining AI decisions, such as feature importance scores or attention mechanisms, have their limitations and may not always provide a complete understanding. Researchers are continually exploring new techniques and frameworks to improve transparency without compromising performance.
Unveiling the Black Box: The Quest for Transparency in AI Decision-Making
Imagine you apply for a loan, and your application is denied. You wonder why, but the explanation is vague, citing a computerized decision. This scenario is common, where AI algorithms assess your creditworthiness based on complex data points, but without clarity on how exactly your application was evaluated. This opacity raises questions about fairness, accountability, and the potential for biases encoded in algorithms.
Transparency in AI decision-making refers to the idea that these systems should provide understandable explanations for their decisions. It’s akin to looking inside the black box to see how the gears turn. This transparency is crucial not only for building trust in AI systems but also for ensuring they operate ethically and fairly.
Think of it like a magic trick: you’re amazed when the magician pulls a rabbit out of the hat, but you’re even more fascinated when they reveal how they did it. Similarly, understanding the inner workings of AI can be both enlightening and empowering. It allows us to scrutinize whether decisions are based on relevant factors or skewed by hidden biases.
Achieving transparency in AI isn’t just a technical challenge; it’s a multidimensional endeavor that involves technology, policy-making, and societal dialogue. Researchers and developers are exploring methods like interpretable machine learning models that provide insights into how AI arrives at its decisions. Meanwhile, policymakers are grappling with the legal and ethical frameworks needed to ensure transparency without compromising proprietary algorithms.
Cracking the Code: Balancing Complexity and Intelligibility in AI Systems
Picture this: you’re chatting with your favorite AI assistant. It’s almost like talking to a friend—until it starts spewing out answers that make your head spin. That’s complexity rearing its sophisticated head. AI systems are designed to process vast amounts of data and make decisions that are, frankly, beyond most of our comprehensions. They’re like those genius kids who can solve quantum physics problems but struggle to tie their shoelaces.
But here’s the catch—if an AI’s responses are too complex, users get frustrated. It’s like getting a gourmet meal when all you wanted was a simple sandwich. This is where intelligibility comes into play. Intelligibility in AI means making sure that these smart systems can communicate their genius in a way that us mere mortals can understand. It’s all about striking that perfect balance.
Think of it as a dance. Complexity and intelligibility need to move in sync, like partners in a perfectly choreographed routine. Too much complexity, and the dance becomes chaotic. Too much focus on simplicity, and the dance loses its flair. AI developers are like dance instructors, tweaking and adjusting until both elements perform in harmony.
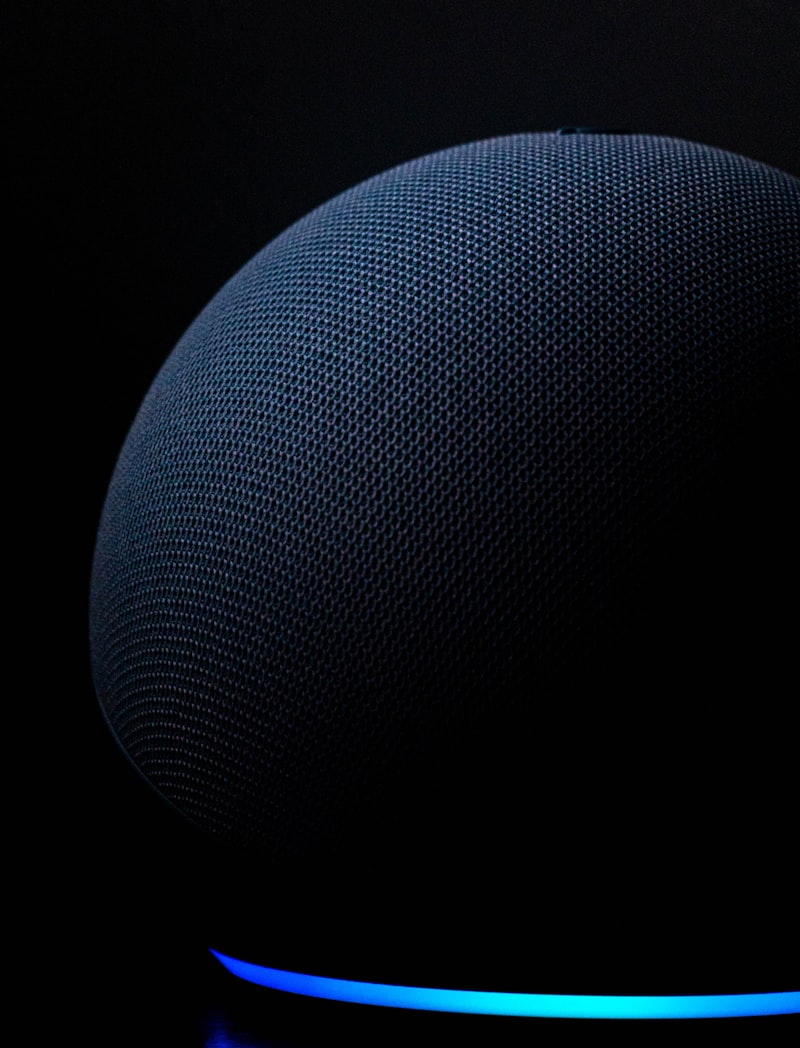
So, how do we achieve this balance? It’s a mix of smart algorithms, user-friendly interfaces, and constant tweaking. Developers are constantly testing and re-testing, ensuring that the AI can handle complex tasks while still speaking our language. It’s a bit like tuning a piano—each key needs to be just right to create a beautiful melody.
In essence, cracking the code of AI means making sure these digital maestros can perform their symphony without missing a beat, while ensuring we can all enjoy the music.
Ethical Dilemmas in Explainable AI: Navigating Bias and Fairness
Imagine AI systems as decision-making assistants, designed to sift through vast amounts of data and make recommendations. Sounds futuristic and efficient, right? But here’s the catch – these systems aren’t infallible. They learn from the data they are fed, and if that data is biased, their decisions can inherit those biases too. It’s like a chef cooking a meal with ingredients that are subtly flawed – the final dish might taste off without you knowing exactly why.
Take hiring practices, for example. AI algorithms are increasingly being used to screen job applications, aiming to find the best candidates objectively. However, if these algorithms are trained on historical hiring data that reflects biases against certain demographics, they might inadvertently perpetuate discrimination. It’s akin to passing down an old family recipe that excludes a key ingredient – the dish loses its richness and potential.
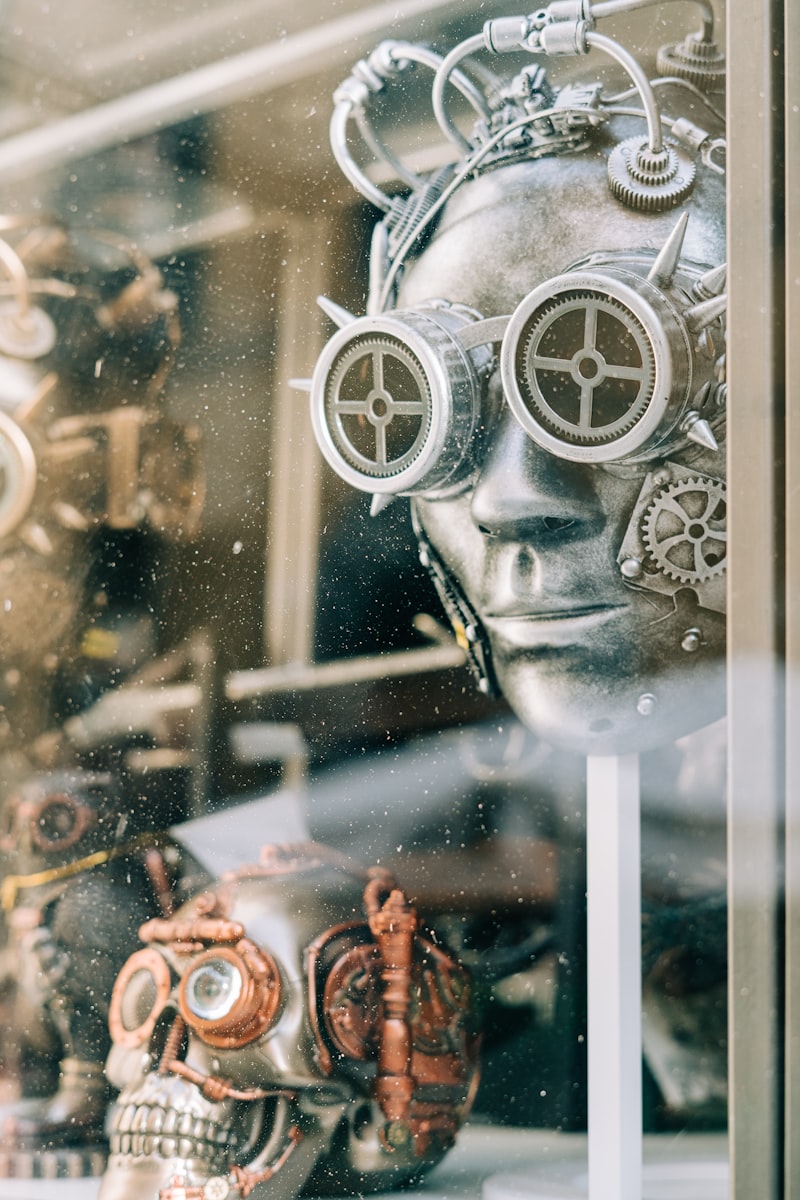
To navigate these ethical minefields, experts advocate for transparency, accountability, and diversity in AI development. Like building a diverse team to taste-test a dish – ensuring varied perspectives can help identify and address biases before they become ingrained in the system.
Beyond Accuracy: The Rise of Intuitive and Interpretable AI Models
Imagine having an AI that not only predicts outcomes with astounding accuracy but also tells you why it made those predictions in a way that makes sense. That’s the promise of intuitive AI models. They aim to bridge the gap between complex algorithms and human understanding, bringing transparency to the forefront of AI development.
One of the key challenges with traditional AI models has been their “black box” nature—where decisions are made without clear explanations. This lack of transparency can be a significant barrier in critical applications such as healthcare diagnostics or autonomous vehicles. Intuitive AI models seek to change that by incorporating design principles that prioritize human interpretability.
Think of it like having a conversation with a friend who not only gives you an answer but also explains their reasoning behind it. This human-like trait in AI not only enhances trust but also opens doors to new possibilities in fields where understanding the rationale behind AI decisions is paramount.
Moreover, these intuitive models are designed to learn from human feedback, much like how we learn from experience. This iterative process of learning and refining models based on real-world interactions helps in creating AI systems that are not only accurate but also adaptable and responsive to changing environments.
Demystifying Algorithms: How to Achieve Explainability Without Sacrificing Performance
Algorithms, those intricate sets of rules and instructions, are the silent choreographers of our digital experiences. They sift through vast amounts of data to recommend movies, predict shopping preferences, or even assess creditworthiness. However, their opaque nature has sparked concerns about bias, accountability, and fairness.
So, how do we achieve explainability in algorithms? It starts with clarity in design. Engineers and data scientists are increasingly adopting methods that prioritize transparency. Instead of relying on complex black-box models, they are favoring simpler, interpretable ones. Imagine an algorithm as a recipe: the clearer the steps, the easier it is to understand why a particular decision was made.
Moreover, using techniques like feature importance and model visualization, experts can shine a light on the inner workings of algorithms. Picture a detective’s magnifying glass revealing clues—the more we see, the more accountable these algorithms become.
Yet, achieving explainability shouldn’t come at the cost of performance. Algorithms need to be both understandable and efficient. Researchers are exploring ways to balance these demands, employing innovative approaches that decode complexity without slowing down processes.
In essence, demystifying algorithms is about empowering users. It’s about lifting the veil on digital decision-making, ensuring that these invisible hands operate with integrity and clarity. As technology evolves, so too must our understanding of how algorithms impact our lives.
Understanding algorithms isn’t just for experts—it’s for anyone who uses technology. By unraveling their mysteries, we pave the way for a more informed and equitable digital future. So, the next time you interact with a recommendation system or a predictive model, remember: clarity is key, and understanding is empowerment.
The Human-AI Interface: Bridging the Gap with Understandable AI
Imagine AI not as a distant, enigmatic force, but as a companion that understands and responds to human needs intuitively. This is the essence of bridging the gap with understandable AI – creating systems that are not only powerful but also approachable, like a helpful guide rather than a complex machine.
Understanding AI shouldn’t feel like deciphering cryptic codes or navigating a labyrinth of technicalities. It’s about making technology accessible and user-friendly, empowering individuals to harness its potential without barriers. Just as a skilled translator makes foreign languages understandable, AI interface designers are crafting intuitive interactions that feel natural to users, regardless of their technical expertise.
Think of AI as a conversation partner rather than a distant expert. Just as you would explain a concept to a friend, understandable AI communicates complex insights in a way that resonates with its users. It’s about clarity and simplicity, ensuring that everyone – from seasoned professionals to curious learners – can benefit from its capabilities.
Moreover, understandable AI fosters trust. When users can easily grasp how AI processes information and makes decisions, they feel more confident in relying on its insights and recommendations. It becomes a tool that augments human intelligence, enhancing productivity and driving innovation across industries.
The Human-AI interface isn’t just about functionality; it’s about empathy and adaptability. Like a skilled teacher tailors lessons to students’ needs, AI interfaces personalize interactions based on user preferences and behaviors. This personalized approach enhances user experience, making technology more responsive and relevant to individual needs.
Legal and Regulatory Challenges in Deploying Transparent AI Systems
Imagine you’ve developed a groundbreaking AI algorithm that can predict market trends with unprecedented accuracy. Exciting, right? But hold on—before unleashing it into the wild, you need to ensure it complies with a myriad of legal frameworks. These regulations aren’t just bureaucratic red tape; they’re guardrails designed to protect consumer rights, ensure fairness, and uphold ethical standards.
One of the foremost challenges is data privacy. Your AI system is hungry for data—lots of it. But feeding it comes with responsibilities. Laws such as the GDPR in Europe or the CCPA in California demand that companies handle personal data with utmost care. Ensuring transparency about what data is collected, how it’s used, and obtaining consent from individuals becomes not just good practice but a legal necessity.
Then there’s the issue of bias. AI systems are only as unbiased as the data they learn from. If your training data inadvertently reflects societal biases, your AI could perpetuate discrimination. Navigating this minefield requires not only technical prowess but also a keen understanding of fairness and accountability.
And let’s not forget accountability itself. When your AI system makes a decision—like recommending a loan application or determining a prison sentence—it must be possible to explain how and why that decision was made. This concept of “algorithmic transparency” isn’t just a buzzword; it’s a cornerstone of building trust in AI technologies.