What Are the Ethical Implications of AI in Criminal Justice?
One of the foremost concerns is fairness. Can AI truly remain impartial, or does it perpetuate existing biases present in historical data? Algorithms trained on biased datasets may inadvertently amplify racial, socioeconomic, or gender biases, leading to unjust outcomes. For instance, studies have shown that AI used in predictive policing often targets marginalized communities disproportionately, perpetuating a cycle of discrimination.
Moreover, transparency becomes critical. How do we ensure that AI decisions are explainable and accountable? Unlike human judges who can justify their decisions, AI operates through complex algorithms that can be opaque to both defendants and the public. The lack of transparency raises concerns about due process and the right to a fair trial.
Privacy is another significant issue. AI systems rely on vast amounts of personal data to make decisions. There are concerns about the collection, storage, and use of this data, especially when it comes to sensitive information about individuals’ lives. How do we balance the benefits of AI with the protection of privacy rights?
Furthermore, the deployment of AI in criminal justice systems raises broader societal questions. Are we comfortable with machines making decisions that have profound impacts on people’s lives? How do we mitigate the risks of automation replacing human judgment and empathy in sensitive legal contexts?
While AI holds great promise in transforming criminal justice systems for the better, its adoption must be accompanied by rigorous ethical considerations. Balancing innovation with ethical principles is crucial to ensuring that AI enhances justice rather than compromising it. As we navigate this evolving landscape, addressing these ethical implications will be paramount in harnessing AI’s potential responsibly.
Algorithmic Justice: Balancing Efficiency with Fairness in AI-Powered Criminal Trials
Imagine a courtroom where algorithms, fueled by vast amounts of data, assist judges in determining sentences. On one hand, these algorithms promise efficiency—they can analyze complex patterns and data points swiftly, potentially reducing the burden on human judges. But on the other hand, they must navigate the complex terrain of human lives and rights without bias or prejudice.
Efficiency, in this context, means quicker decision-making processes. Algorithms can process immense datasets in seconds, identifying trends and patterns that might not be immediately apparent to a human eye. This speed could mean faster resolutions for cases, reducing backlog and improving overall judicial efficiency.
However, the real challenge lies in balancing this efficiency with fairness. Algorithms, while powerful, are only as unbiased as the data they are trained on. If historical data reflects biases in arrests, sentencing, or policing practices, the algorithms could perpetuate these biases, leading to unfair outcomes for certain demographic groups.
Think of it this way: an algorithm is like a magnifying glass—it can reveal intricate details and correlations, but it can also amplify existing biases if not carefully calibrated. Just as a magnifying glass needs to be held steady to focus on the truth, algorithms must be meticulously designed and constantly monitored to ensure they uphold principles of justice and equity.
Achieving Algorithmic Justice requires a delicate balance. It demands transparency in algorithmic decision-making processes, rigorous testing for biases, and continuous refinement based on real-world outcomes. Only then can these AI systems truly serve as tools for justice, augmenting human judgment without compromising on the core values of fairness and equality.
Bias Behind Bars: Unveiling the Ethical Dilemmas of AI in Sentencing
One of the foremost ethical dilemmas revolves around the inherent biases within AI algorithms themselves. These algorithms, though designed to be impartial, can inadvertently perpetuate biases present in the data used to train them. For instance, if historical sentencing data reflects biases against certain demographics, such as minorities or economically disadvantaged groups, AI algorithms trained on this data might replicate or even amplify these biases. This raises profound questions about fairness and justice in sentencing practices.
Moreover, the opacity of AI decision-making poses significant challenges. Unlike human judges whose reasoning can be scrutinized and appealed, AI algorithms operate as black boxes, making it difficult to understand how they arrive at their decisions. This lack of transparency not only undermines accountability but also compromises the rights of defendants to understand and challenge the basis of their sentencing.
Another critical concern is the potential for AI to entrench existing disparities in sentencing outcomes. By relying on historical data that reflects systemic inequalities, AI algorithms risk perpetuating unequal treatment under the guise of impartiality. This not only threatens the principles of justice but also erodes public trust in the fairness of judicial systems.
Furthermore, the deployment of AI in sentencing decisions raises profound philosophical questions about the nature of justice itself. Can true justice be achieved through algorithms devoid of human empathy and contextual understanding? Or does justice demand a nuanced, human-centered approach that considers the complexities of each individual case?
As we navigate the evolving landscape of AI in sentencing, it becomes imperative to address these ethical dilemmas with careful consideration and vigilance. While AI holds promise in enhancing judicial efficiency, its integration must be accompanied by robust safeguards to mitigate biases, ensure transparency, and uphold the principles of fairness and equity in sentencing. The path forward requires a delicate balance between harnessing technological advancements and preserving the fundamental tenets of justice that form the bedrock of our legal systems.
Data Over Judgment: How AI Decisions in Criminal Justice Challenge Human Rights
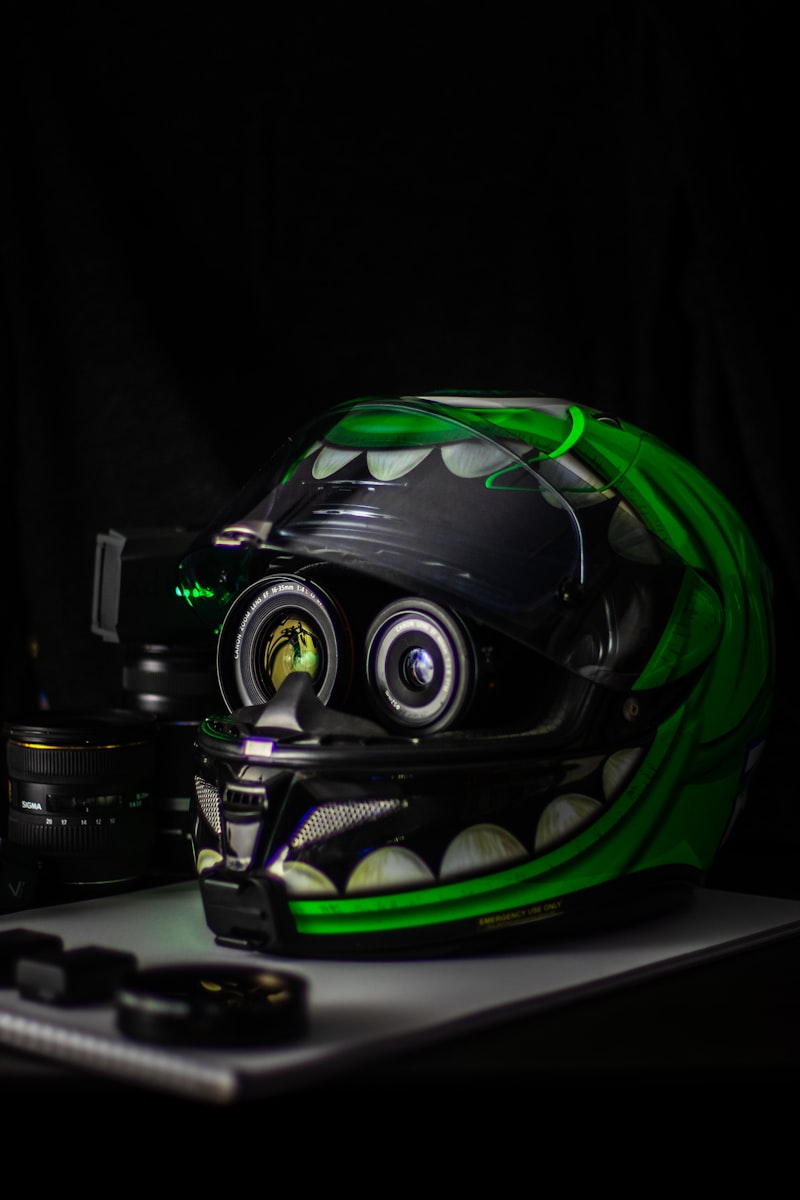
However, the use of AI in criminal justice raises critical questions about its reliability and ethical implications. Can an algorithm truly understand the complexities of human behavior and the nuances of each individual case? Critics argue that AI systems are only as good as the data they are trained on, and if that data reflects biases or inaccuracies, it can perpetuate and even exacerbate existing injustices.
Moreover, there is the issue of transparency and accountability. Unlike human judges who can explain their reasoning, AI algorithms often operate as black boxes, making it difficult to understand how they arrive at their decisions. This lack of transparency can erode trust in the justice system and deny individuals the opportunity to challenge decisions that affect their lives.
Consider the analogy of a compass versus a GPS. While a compass guides based on magnetic fields, a GPS relies on satellite data and algorithms to navigate. The GPS may offer more precise directions, but if the underlying data is flawed, it can lead you astray. Similarly, AI in criminal justice must navigate carefully to ensure it serves justice without compromising human rights.
Transparency vs. Efficiency: The Moral Crossroads of AI Adoption in Law Enforcement
Imagine a future where AI-powered systems can predict crime patterns, analyze surveillance footage, and even assess the likelihood of individuals committing offenses. On one hand, this promises unprecedented efficiency in crime prevention and investigation. AI can process vast amounts of data at speeds beyond human capability, potentially identifying trends and anomalies that human analysts might overlook. This efficiency could lead to quicker response times, more accurate decision-making, and ultimately, safer communities.
However, the pursuit of efficiency through AI raises significant concerns about transparency and accountability. Unlike human officers whose decision-making processes are, ideally, subject to scrutiny and oversight, AI algorithms often operate as black boxes. They process data using complex algorithms that even their creators might not fully understand, making it challenging to explain how certain decisions or predictions are reached. This lack of transparency can erode trust in law enforcement agencies and raise questions about fairness and bias in AI-driven decision-making.
Moreover, the moral implications of AI adoption in law enforcement are profound. Should algorithms be trusted to make decisions that affect individuals’ lives, such as predicting whether someone is likely to commit a crime? How can we ensure that AI systems do not perpetuate or amplify existing biases in policing practices?
In navigating this moral crossroads, stakeholders must carefully balance the potential benefits of AI in enhancing law enforcement efficiency with the imperative of maintaining transparency and ethical standards. Perhaps the key lies in developing AI systems that are not only efficient but also transparent and accountable. This involves designing algorithms that can be audited, ensuring diverse representation in AI development teams, and establishing robust oversight mechanisms to monitor AI deployment in real-world scenarios.
Ultimately, the debate between transparency and efficiency in AI adoption reflects broader societal questions about the responsible use of technology in upholding justice and fairness. As we continue to harness the power of AI in law enforcement, addressing these ethical considerations will be crucial in shaping a future where technology serves justice while upholding fundamental moral values.
Preventing Prejudice: Can AI Algorithms Truly Achieve Impartiality in Legal Proceedings?
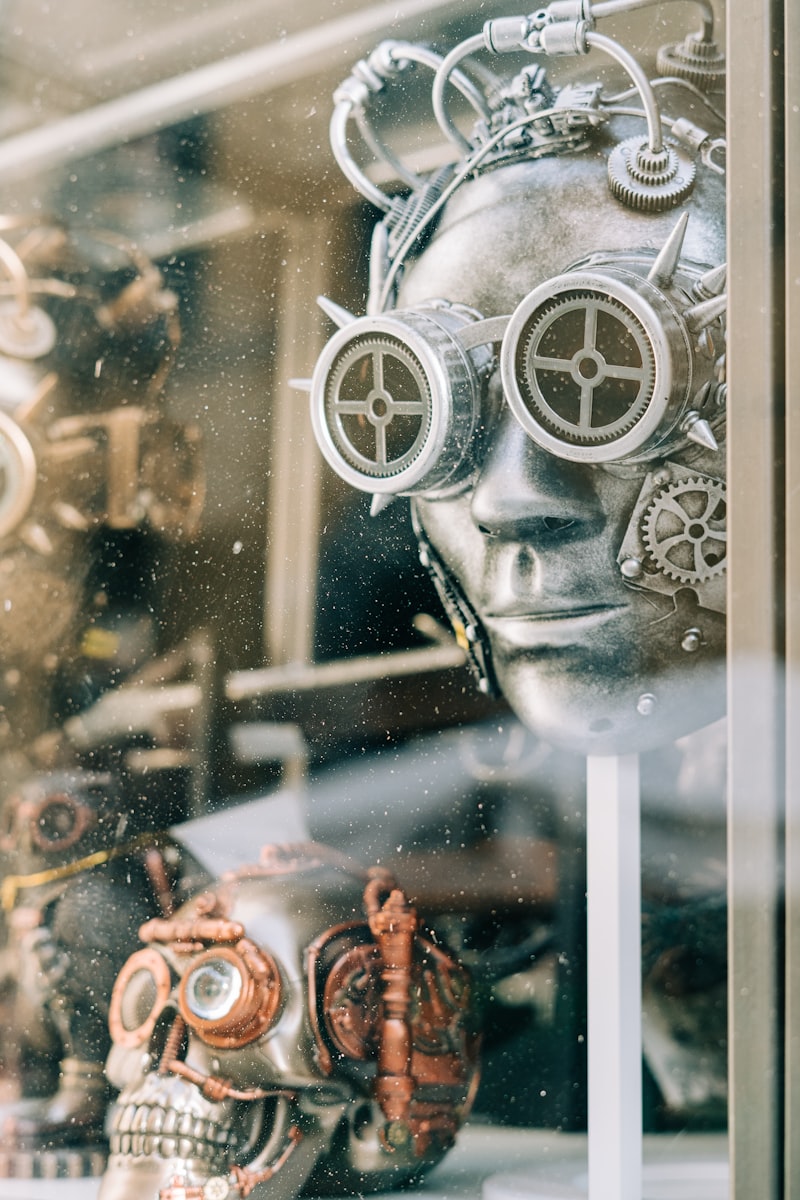
Imagine AI as a digital judge, devoid of personal biases or preconceptions. It evaluates cases based solely on the facts presented, devoid of emotional influences or external pressures. This ability stems from its capacity to process vast amounts of data, identifying patterns and correlations that human judges might overlook.
However, achieving true impartiality isn’t just about processing data; it’s about interpreting it accurately. AI algorithms are designed to continuously learn and adapt, refining their decision-making processes with each case they analyze. This iterative learning mimics human cognitive processes but operates on a scale and speed that far surpasses our biological capabilities.
But can AI truly eliminate all forms of bias? That’s the critical question. While AI algorithms are programmed to be neutral, the data they learn from may reflect societal biases inherent in human behavior and historical precedents. For instance, if historical legal data shows a disproportionate number of rulings against a specific demographic, AI might inadvertently perpetuate these biases if not properly corrected.
To address this challenge, developers are implementing techniques like bias detection algorithms and data sanitization protocols. These methods aim to identify and mitigate biases within the training data, ensuring that AI algorithms make decisions that are fair and impartial across all demographics.
While AI algorithms hold great promise in enhancing impartiality within legal proceedings, achieving true neutrality requires ongoing scrutiny and refinement. As technology evolves, so too must our ethical frameworks and regulatory standards. The journey towards impartiality in the courtroom is ongoing, driven by a commitment to fairness and justice in an increasingly digital age.